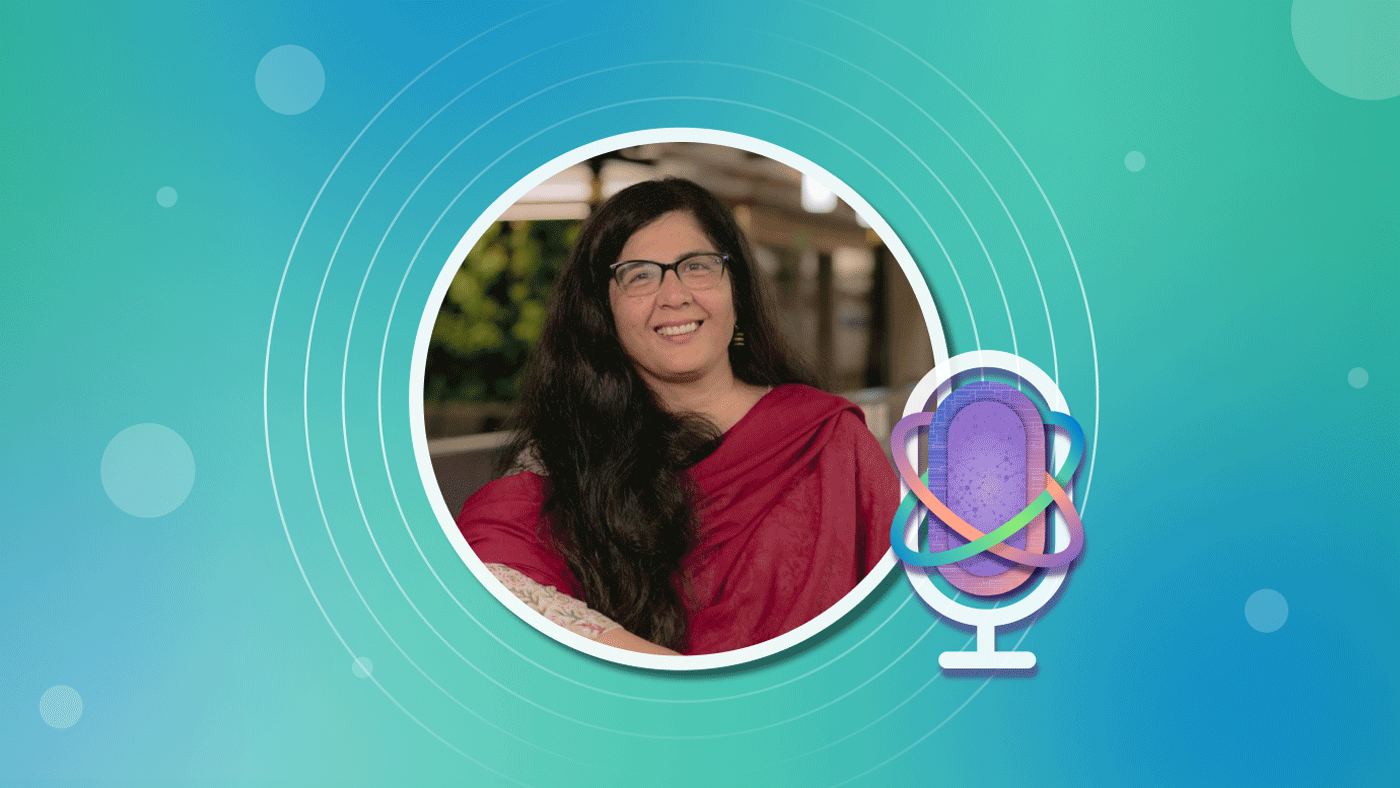
Behind every emerging technology is a great idea propelling it forward. In the new Microsoft Research Podcast series, Ideas, members of the research community at Microsoft discuss the beliefs that animate their research, the experiences and thinkers that inform it, and the positive human impact it targets.
In this episode, host Gretchen Huizinga talks with Principal Researcher Kalika Bali. Inspired by an early vision of “talking computers” and a subsequent career in linguistics, Bali has spent the last two decades bringing the two together. Aided by recent advances in large language models and motivated by her belief that everyone should have access to AI in their own language, Bali and her teams are building language technology applications that they hope will bring the benefits of generative AI to under-resourced and underserved language communities around the world.
Learn more:
- The State and Fate of Linguistic Diversity and Inclusion in the NLP World
Publication, July 2020 - Project VeLLM
Project page - Kahani: Visual Storytelling
Project page - Kahani: Visual Storytelling through Culturally Nuanced Images
Microsoft Research Forum | Episode 1, January 2024 - Teachers in India help Microsoft Research design AI tool for creating great classroom content
Microsoft Research blog, October 2023 - Digital Labor: Project Karya
Project page - Village by village, creating the building blocks for AI tools with work that also educates (opens in new tab)
Microsoft Source Asia blog, February 2024
Subscribe to the Microsoft Research Podcast:
Transcript
[TEASER]
[MUSIC PLAYS UNDER DIALOGUE]
KALIKA BALI: I do think, in some sense, the pushback that I got for my idea makes me think it was outrageous. I didn’t think it was outrageous at all at that time! I thought it was a very reasonable idea! But there was a very solid pushback and not just from your colleagues. You know, for researchers, publishing papers is important! No one would publish a paper which focused only on, say, Indian languages or low-resource languages. We’ve come a very long way even in the research community on that, right. We kept pushing, pushing, pushing! And now there are tracks, there are workshops, there are conferences which are devoted to multilingual and low-resource languages.
[TEASER ENDS]
GRETCHEN HUIZINGA: You’re listening to Ideas, a Microsoft Research Podcast that dives deep into the world of technology research and the profound questions behind the code. I’m Dr. Gretchen Huizinga. In this series, we’ll explore the technologies that are shaping our future and the big ideas that propel them forward.
[MUSIC FADES]
I’m excited to be live in the booth today with Kalika Bali, a principal researcher at Microsoft Research India. Kalika is working on language technologies that she hopes will bring the benefits of generative AI to under-resourced and underserved language communities around the world. Kalika, it’s a pleasure to speak with you today. Welcome to Ideas!
KALIKA BALI: Thank you. Thank you, Gretchen. Thank you for having me.
HUIZINGA: So before we dive in on the big ideas behind Kalika Bali’s research, let’s talk about you for a second. Tell us about your “origin story,” as it were, and if there is one, what “big idea” or animating “what if?” captured your imagination and inspired you to do what you’re doing today?
BALI: So, you know, I’m a great reader. I started reading well before I was taught in school how to read, and I loved science fiction. I come from a family where reading was very much a part of our everyday lives. My dad was a journalist, and I had read a lot of science fiction growing up, and I also saw a lot of science fiction, you know, movies … Star Trek … everything that I could get hold of in India. And I remember watching 2001: Space Odyssey. And there was this HAL that spoke. He actually communicated that he was a computer. And I was just so struck by it. I was like, this is so cool! You know, here are computers that can talk! Now, how cool would that be if it would happen in real life? I was not at all aware of what was happening in speech technology, whether it was possible or not possible, but that’s something that really got me into it. I’ve always, like, kind of, been very curious about languages and how they work and, you know, how people use different things in languages to express not just meaning, not just communicating, but you know expressing themselves, really. And so I think it’s a combination of HAL and this curiosity I had about the various ways in which people use languages that got me into what I’m doing now.
HUIZINGA: OK. So that’s an interesting path, and I want to go into that just a little bit, but let me anchor this: how old were you when you saw this talking computer?
BALI: Oh, I was in my early teens.
HUIZINGA: OK. And so at that time, did you have any conception that … ?
BALI: No. You know, there weren’t computers around me when I was growing up. We saw, you know, some at school, you know, people coded in BASIC …
HUIZINGA: Right?
BALI: And we heard about them a lot, but I hadn’t seen one since I was in high school.
HUIZINGA: OK. So there’s this inception moment, an aha moment, of that little spark and then you kind of drifted away from the computer side of it, and what … tell us about how you went from there to that!
BALI: So that, that’s actually a very funny story because I actually wanted to study chemistry. I was really fascinated by how these, you know, molecular parts rotate around each other and, you know, we can’t even tell where an electron is, etc. It sounded, like, really fun and cool. So I actually studied chemistry, but then I was actually going to pick up the admission form for my sister, who wanted to study in this university, and … or, no, she wanted to take an exam for her master’s. And I went there. I picked up the form, and I said, this is a cool place. I would love to study here! And then I started looking at everything like, you know, what can I apply for here? And something called linguistics came up, and I had no idea what linguistics was. So I went to the British Library, got like a thin book on introduction to linguistics, and it sounded fun! And I took the exam. And then, as they say, that was history. Then I just got into it.
HUIZINGA: OK. I mean, so much has happened in between then and now, and I think we’ll kind of get there in … but I do want you to connect the larger dot from how you got from linguistics to Microsoft Research [LAUGHTER] as a computer scientist.
BALI: So I actually started teaching at the University of South Pacific as a linguistics faculty in Fiji. And I was very interested in acoustics of speech sounds, etc., etc. That’s what I was teaching. And then there was a speech company in Belgium that was looking to start some work in Indian languages, and they contacted me, and at that time, you needed people who knew about languages to build language technology, especially people who knew about phonetics, acoustics, for speech technology. And that’s how I got into it. And then, you know, I just went from startups to companies and then Microsoft Research, 18 years ago, almost 18 years ago.
HUIZINGA: Wow. OK. I would love to actually talk to you about all that time. But we don’t have time because I have a lot more things to talk to you about, technology-wise. But I do want to know, you know, how would you describe the ideas behind your overarching research philosophy, and who are your influences, as they say in the rock-and-roll world? [LAUGHTER] Who inspired you? Real-life person, scientist or not, besides, HAL 9000, who’s fictional, and any seminal papers that, sort of, got you interested in that along the way?
BALI: So since I was really into speech, Ken Stevens—who was a professor, who sadly is no longer with us anymore, at MIT—was a big influence. He, kind of, had this whole idea of how speech is produced. And, you know, the first time I was exposed to the whole idea of the mathematics behind the speech, and I think he influenced me a lot on the speech side of things. For the language side of things, you know, my professor in India Professor Anvita Abbi—you know, she’s a Padma Shri, like, she’s been awarded by the Indian government for her work in, you know, very obscure, endangered languages—you know, she kind of gave me a feel for what languages are, and why they are important, and why it’s important to save them and not let them die away.
HUIZINGA: Right.
BALI: So I think I would say both of them. But what really got me into wanting to work with Indian language technology in a big way was I was working in Belgium, I was working in London, and I saw the beginning of how technology is, kind of, you know, making things easier, exciting; there’s cool technology available for English, for French, for German … But in a country like India, it was more about giving access to people who have no access, right? It actually mattered, because here are people who may not be very literate and therefore not be able to use technology in the way we know it, but they can talk.
HUIZINGA: Right.
BALI: And they can speak, and they should be able to access technology by doing that.
HUIZINGA: Right. OK. So just real quickly, that was then. What have you seen change in that time, and how profoundly have the ideas evolved?
BALI: So just from pure methodology and what’s possible, you know, I have seen it all. When I started working in language technology, mainly for Indian languages, but even for other languages, it was all a rule-based system. So everybody had to create all these rules that then were, you know, responsible for building or like making that technology work. But then, just at that time, you know, all the statistical systems and methodologies came into being. So we had hidden Markov models, you know, doing their thing in speech, and it was all about a lot of data. But that data still had to be procured in a certain way, labeled, annotated. It was still a very long and resource-intensive process. Now, with generative AI, the thing that I am excited about is, we have a very powerful tool, right?
HUIZINGA: Mm-hmm.
BALI: And, yes, it requires a lot of data, but it can learn also; you know, we can fine-tune stuff on smaller datasets …
HUIZINGA: Yeah …
BALI: … to work for, you know, relevant things. So it’s not going to take me years and years and years to first procure the data, then have it tagged for part of speech … then, you know, have it tagged for sentiment, have it tagged for this, have it tagged for that, and then, only can I think of building anything.
HUIZINGA: Right.
BALI: So it just shortens that timeline so much, and it’s very exciting.
HUIZINGA: Right. As an ex-English teacher—which I don’t think there is such a thing as an ex-English teacher; you’re always silently correcting someone’s grammar! [LAUGHTER]—just what you said about tagging parts of speech as what they are, right? And that, I used to teach that. And then you start to think, how would you translate that for a machine? So fascinating. So, Kalika, you have said that your choice of career was accidental—and you’ve alluded to the, sort of, the fortuitous things that happened along the way—but that linguistics is one subject that goes from absolute science to absolute philosophy. Can you unpack that a little bit more and how this idea impacted your work in language technology?
BALI: Yeah. So, so if you think about it, you know, language has a physical aspect, right. We move our various speech organs in a certain way. Our ears are constructed in a certain way. There is a physics of it where, when I speak, there are sound waves, right, which are going into your ear, and that’s being interpreted. So, you know, if you think about that, that’s like an absolute science behind it, right? But then, when you come to the structure of language, you know, the syntax, like you’re an English teacher, so you know this really well, that you know, there’s semantics; there’s, you know, morphology, how our words form, how our sentences form. And that’s like a very abstract kind of method that allows us to put, you know, meaningful sentences out there, right?
HUIZINGA: Right …
BALI: But then there’s this other part of how language works in society, right. The way I talk to my mother would be probably very different to the way I’m talking to you, would be very different from the way I talk to my friends, at a very basic level, right? The way, in India, I would greet someone older to me would be very different from the way I would greet somebody here, because here it’s like much less formal and that, you know, age hierarchy is probably less? If I did the same thing in India, I would be considered the rudest creature ever. [LAUGHS] So … and then, you know, you go into the whole philosophy—psycholinguistics part. What happens in our brains, you know, when we are speaking? Because language is controlled by various parts of our brain, right. And then, you go to the pure philosophy part, like why? How does language even occur? Why do we name things the way we name things? You know, why do we have a language of thought? You know, what language are we thinking in? [LAUGHTER]
HUIZINGA: Right.
BALI: So, so it really does cover the entire gamut of language …
HUIZINGA: Yeah, yeah, yeah …
BALI: … like from science to philosophy.
HUIZINGA: Yeah, as I said before, when we were talking out there, my mother-in-law was from Holland, and every time she did math or adding, she would do it in Dutch, which—she’d be speaking in English and then she’d go over here and count in Dutch out loud. And it’s like, yeah, your brain switches back and forth. This is so exciting to me. I had no idea how much I would love this podcast! So, much of your research is centered on this big idea called “design thinking,” and it’s got a whole discipline in universities around the world. And you’ve talked about using something you call the 4D process for your work. Could you explain that process, and how it plays out in the research you do with the communities you serve?
BALI: Yeah, so we’ve kind of adapted this. My ex-colleague Monojit Choudhury and I, kind of, came up with this whole thing about 4D thinking, which is essentially discover, design, develop and deploy, right. And when we are working with, especially with, marginalized or low-resource-language communities, the very basic thing we have to do is discover, because we cannot go with, you know, our own ideas and perceptions about what is required. And I can give you a very good example of this, right. You know, most of us, as researchers and technologists, when we think of language technology, we are thinking about machine translation; we’re thinking about speech recognition; we are thinking about state-of-the-art technology. And here we were talking to a community that spoke the language Idu Mishmi, which is a very small community in northeast of India. And we were talking about, you know, we can do this, we can do that. And they just turned to us and said, what we really want is a mobile digital dictionary! [LAUGHS]
HUIZINGA: Wow. Yeah …
BALI: Right? And, you know, if you don’t talk, if you don’t observe, if you are not open to what the community’s needs might be, then you’ll miss that, right. You’ll miss the real thing that will make a difference to that community. So that’s the discover part. The design part, again, you have to design with the community. You cannot go and design a system that they are unable to use properly, right. And again, another very good example, one of the people I know, you know, he gave me this very good example of why you have to think, even at the architecture level when you’re designing such things, is like a lot of applications in India and around the world require your telephone number for verification. Now, for women, it might be a safety issue. They might not want to give their telephone number. Or in India, many women might not even have a telephone, like a mobile number, right. So how do you think of other ways in which they can verify, right? And so that’s the design part. The develop and the deploy part, kind of, go hand in hand, because I think it’s a very iterative process. You develop quickly, you put it out there, allow it to fail and, you know …
HUIZINGA: Mm-hmm. Iterate …
BALI: Iterate. So that’s like the, kind of, design thinking that we have.
HUIZINGA: Yeah, I see that happening in accessibility technology areas, too, as well as language …
BALI: Yeah, and, you know, working with the communities, very quickly, you become really humble.
HUIZINGA: Sure.
BALI: There’s a lot of humility in me now. Though I have progressed in my career and, you know, supposedly become wiser, I am much more humble about what I know and what I can do than I was when I started off, you know.
HUIZINGA: I love that. Well, one thing I want to talk to you about that has intrigued me, there’s a thing that happens in India where you mix languages …
BALI: Yes!
HUIZINGA: You speak both Hindi and English at the same time, and you think, oh, you speak English, but it’s like, no, there’s words I don’t understand in that. What do you call that, and how did that drive your interest? I mean, that was kind of an early-on kind of thing in your work, right? Talk about that.
BALI: So that’s called code-mixing or code-switching. The only linguistic difference is code-mixing happens within a sentence, and code-switching means one sentence in one language and another.
HUIZINGA: Oh, really?
BALI: Yeah. So … but this is, like, not just India. This is a very, very common feature of multilingual societies all over the world. So it’s not multilingual individuals, but at the societal level, when you have multilingualism, then, you know, this is a marker of multilingualism. But code-mixing particularly means that you have to be fluent in both languages to actually code-mix, right. You have to have a certain amount of fluency in both languages. And there are various reasons why people do this. You know, it’s been studied by psychologists and linguists for a long time. And for most people like me, multilingual people, that’s the language we dream in, we think about. [LAUGHTER] That’s the language we talk to our siblings and friends in, right. And for us, it’s, like, just natural. We just keep …
HUIZINGA: Mixing …
BALI: … flipping between the two languages for a variety of reasons. We might do it for emphasis; we might do it for humor. We might just decide, OK, I’m going to pick this from this … the brain decides I’m going to pick this from this language …
HUIZINGA: Sure.
BALI: … and this … So the reason we got interested in, like, looking into code-mixing was that when we are saying that we want humans to be able to interact with machines in their most natural language, then by some estimates, half the world speaks like this!
HUIZINGA: Right.
BALI: So we have to be able to understand exactly how they speak and, you know, be able to process and understand their language, which is code-mixed …
HUIZINGA: Sure. Well, it seems like the human brain can pick this up and process it fairly quickly and easily, especially if it knows many languages. For a machine, it would be much more difficult?
BALI: It is. So initially, it was really difficult because, you know, the way we created systems was one language at a time …
HUIZINGA: Right!
BALI: … right. And it’s not about having an English engine and a Hindi engine available. It doesn’t work that way.
HUIZINGA: No!
BALI: So you’d really need something that, you know, is able to tackle the languages together. And in some theories, this is almost considered a language of its own because it’s not like you’re randomly mixing. There is a structure to …
HUIZINGA: Oh, is there?
BALI: Yeah. Where you can, where you can’t …
HUIZINGA: Gotcha.
BALI: You know, so there is a structure or grammar, you can say, of code-mixing. So we went after that. We, kind of, created tools which could generate grammatically viable code-mixed sentences given parallel data, etc.
HUIZINGA: That’s awesome. Amazing.
BALI: So, yeah, it takes effort to do it. But again, right now, because the generative AI models have at their disposal, you know, so many languages and at least, like, theoretically can work in many, many, many languages, you know, code-mixing might be an easier problem to solve right now.
HUIZINGA: Right. OK. So we’re talking mostly about widely used languages, and you’re very concerned right now on this idea of low-resource languages. So unpack what you mean by low-resource, and what’s missing from the communities that speak those languages?
BALI: Yeah. So when we say low-resource languages, we typically mean that languages do not have, say, digital resources, linguistic resources, language resources, that would enable technology building. It doesn’t mean that the communities themselves are impoverished in culture or linguistic richness, etc., right. But the reason why these communities do not have a lot of language resources, linguistic resources, digital resources, most of the time, it is because they are also marginalized in other ways … social and economic marginalization.
HUIZINGA: Right.
BALI: And these are … if you look at them, they’re not ti—I mean, of course, some of them are tiny, but when we say low-resource communities, we are talking about really big numbers.
HUIZINGA: Oh, really?
BALI: Yeah. So one of the languages that I have worked with—language communities that I’ve worked with—speak a language called Gondi, which is like a Dravidian language that is spoken in … like a South Indian language that is spoken in north, central-north area. It’s a tribal language, and it’s got around three million speakers.
HUIZINGA: Oh, wow!
BALI: Yeah. That’s like more than Welsh, …
HUIZINGA: Yeah! [LAUGHS]
BALI: … right? But because socio-politically, they have been—or economically, they have been marginalized, they do not have the resources to build technologies. And, you know, when we say empower everyone and we only empower the top tier, I don’t think we fulfill our ambition to empower everyone. And like I said earlier, for these communities, all the technology that we have, digital tools that we have access to, they really matter for them. So, for example, you know, a lot of government schemes or the forest reserve laws are provided, say, in Hindi. If they are provided in Gondi, these people have a real idea of what they can do.
HUIZINGA: Yeah. … Sure.
BALI: Similarly, for education, you know, there are books and books and books in Hindi. There’s no book available for Gondi. So how is the next generation even going to learn the language?
HUIZINGA: Right.
BALI: And there are many, many languages which are low resource. In fact, you know, we did a study sometime in 2020, I think, we published this paper on linguistic diversity, and there we saw that, you know, we divided languages in five categories, and the top most which have all the resources to build every possible technology have only five languages, right. And more than half of the world’s languages are at the bottom. So it is a big problem.
HUIZINGA: Yeah. Let’s talk about some of the specific technologies you’re working on. And I want to go from platform to project because you’ve got a big idea in a platform you call VeLLM. Talk about that.
BALI: So VeLLM, which actually means jaggery—the sweet, sugary jaggery—in Tamil, one of the languages in India …
HUIZINGA: Let me, let me interject that it’s not vellum like the paper, or what you’re talking about. It’s capital V, little e, and then LLM, which stands for large language model?
BALI: So universal, the “V” comes from there. Empowerment, “e” comes from there. Through large language models …
HUIZINGA: Got it. OK. But you shortened it to VeLLM.
BALI: Yeah.
HUIZINGA: OK.
BALI: So, so the thing with VeLLM is that a bunch of us got together just when this whole GPT was released, etc. We have a very strong group that works on technologies for empowerment in the India lab, Microsoft Research India. And we got together to see what it is that we can do now that we have access to such a strong and powerful tool. And we started thinking of the work that we’ve been doing, which is to, you know, build these technologies for specific areas and specific languages, specific demographies. So we, kind of, put all that knowledge and all that experience we had and thought of like, how can we scale that, really, across everything that we do? So VeLLM, at its base, you know, takes a GPT-like LLM, you know, as a horizontal across everything. On top of it, we have again, horizontals of machine learning, of multilingual tools and processes, which allow us to take the outputs from, say, GPT-like things and adapt it to different languages or, you know, some different kind of domain, etc. And then we have verticals on top of it, which allow people to build specific applications.
HUIZINGA: Let me just go back and say GPT … I think most of our audience will know that that stands for generative pretrained transformer models. But just so we have that for anyone who doesn’t know, let’s anchor that. So VeLLM basically was an enabling platform …
BALI: Yes.
HUIZINGA: … on which to build specific technologies that would solve problems in a vertical application.
BALI: Yes. Yes. And because it’s a platform, we’re also working on tools that are needed across domains …
HUIZINGA: Oh, interesting.
BALI: … as well as tools that are needed for specific domains.
HUIZINGA: OK, so let’s talk about some of the specifics because we could get into the weeds on the tools that everybody needs, but I like the ideas that you’re working on and the specific needs that you’re meeting, the felt-need thing that gets an idea going. So talk about this project that you’ve worked on called Kahani. Could you explain what that is, and how it works? It’s really interesting to me.
BALI: So Kahani, actually, is about storytelling, culturally appropriate storytelling, with spectacular images, as well as like textual story.
HUIZINGA: So visual storytelling?
BALI: Visual storytelling with the text. So this actually started when my colleague Sameer Segal, he was trying to use generative AI to create stories for his daughter, and he discovered that, you know, things are not very culturally appropriate! So I’ll give an example that, you know, if you want to take Frozen and take it to, like, the south Indian state of Kerala, you’ll have the beaches of Kerala, you’ll have even have the coconut trees, but then you will have this blond princess in a princess gown …
HUIZINGA: Sure …
BALI: … who’s there, right? So that’s where we started discussing this, and we, kind of, started talking about, how can we create visuals that are anchored on text of a story that’s culturally appropriate? So when we’re talking about, say, Little Red Riding Hood, if we ask the generative AI model, OK, that I want the story of Little Red Riding Hood but in an Indian context, it does a fantastic job. It actually gives you a very nice story, which, you know, just reflects the Red Riding Hood story into an Indian context. But the images don’t really …
HUIZINGA: Match … [LAUGHTER]
BALI: … Match at all. So that’s where the whole Kahani thing started. And we did a hackathon project on it. And then a lot of people got interested. It’s an ongoing project, so I won’t say that it’s out there yet, but we are very excited about it, but because think of it, we can actually create stories for children, you know, which is what we started with, but we can create so much more media, so much more culturally appropriate storytelling, which is not necessarily targeted at children.
HUIZINGA: Yeah, yeah.
BALI: So that’s what Kahani is about.
HUIZINGA: OK. And I saw a demo of it that your colleague did for Research Forum here, and there was an image of a girl—it was beautiful—and then there was a mask of some kind or a … what was that?
BALI: So the mask is called Nazar Battu, which is actually, you have these masks which are supposed to drive away the evil eye. So that’s what the mask was about. It’s a very Indian thing. You know, when you build a nice house, you put one on top of it so that the envious glances are, like, kept at bay. So, yeah, so that’s what it was.
HUIZINGA: And was there some issue of the generative AI not really understanding what that was?
BALI: No, it didn’t understand what it was.
HUIZINGA: So then can you fix that and make it more culturally aware?
BALI: So that’s what we are trying to do for the image thing. So we have another project on culture awareness where we are looking at understanding how much generative AI knows about other cultures.
HUIZINGA: Interesting.
BALI: So that’s a simultaneous project that’s happening. But in Kahani, a lot of it is, like, trying to get reference images, you know …
HUIZINGA: Yeah. … Into the system?
BALI: Into the system …
HUIZINGA: Gotcha …
BALI: … and trying to anchor on that.
HUIZINGA: Mmmm. So—and we’re not going to talk about that project, I don’t think—but … how do you assess whether an AI knows? By just asking it? By prompting and seeing what happens?
BALI: Yeah, yeah, yeah. So in another project, what we did was, we asked humans to play a game to get cultural artifacts from them. The problem with asking humans what cultural artifacts are important to them is we don’t think of like things as culture, right. [LAUGHS] This is food!
HUIZINGA: It’s just who we are!
BALI: This is my food. Like, you know, it’s not a culturally important artifact. This is how I greet my parents. It’s not like culturally …
HUIZINGA: So it’s just like fish swimming in water. You don’t see the water.
BALI: Exactly. So we gamified this thing, and we were able to get certain cultural artifacts, and we tried to get generative AI models to tell us about the same artifacts. And it didn’t do too well … [LAUGHS]
HUIZINGA: But that’s why it’s research!
BALI: Yes!
HUIZINGA: You try, you iterate, you try again … cool. As I mentioned earlier, I was a high school English teacher and an English major. I’m not correcting your grammar because it’s fantastic.
BALI: Thank you.
HUIZINGA: But as a former educator, one of the projects I felt was really compelling that you’re working on is called Shiksha. It’s a copilot in education. Tell our audience about this.
BALI: So this is actually our proof of concept for the VeLLM platform. Since almost all of us were interested in education, we decided to go for education as the first use case that we’re going to work on. And actually, it was a considered decision to go target teachers instead of students. I mean, you must have seen a lot of work being done on taking generative AI to students, right. But we feel that, you know, teachers are necessary to teach because they’re not just giving you information about the subject. They’re giving you skills to learn, which hopefully will stay with you for a lifetime, right. And if we enable teachers, they will enable so many hundreds of students. One teacher can enable thousands of students, right, over her career. So instead of, like, going and targeting students, if we make it possible for teachers to do their jobs more effectively or, like, you know, help them get over the problems they have, then we are actually creating an ecosystem where things will scale really fast, really quickly. And in India, you know, this is especially true because the government has actually come up with some digital resources for teachers to use, but there’s a lot more that can be done. So we interviewed about a hundred-plus teachers across different parts of the country. And this is the, you know, discover part.
HUIZINGA: Yeah!
BALI: And we found out that lesson plans are a big headache! [LAUGHS]
HUIZINGA: Yes, they are! Can confirm!
BALI: Yeah. And they spend a lot of time doing lesson plans because they’re required to create a lesson plan for every class they teach …
HUIZINGA: Sure. With learning outcomes …
BALI: Exactly.
HUIZINGA: All of it.
BALI: All of it. So that’s where we, you know, zeroed in on—how to make it easier for teachers to create lesson plans. And that’s what the Shiksha project is about. You know, there is an enrollment process where the teachers say what subject they’re teaching, what classes they’re teaching, what boards, because there are different boards of education …
HUIZINGA: Right …
BALI: … which have different syllabus. So all that. But after that, it takes less than seven minutes for a teacher to create an entire lesson plan for a particular topic. You know, class assignments, class activities, home assignments, homework—everything! Like the whole thing in seven minutes! And these teachers have the ability to go and correct it. Like, it’s an interactive thing. So, you know, they might say, I think this activity is too difficult for my students.
HUIZINGA: Yeah …
BALI: Can I have, like, an easier one? Or, can I change this to this? So it allows them to interactively personalize, modify the plan that’s put out. And I find that really exciting. And we’ve tested this with the Sikshana Foundation, which works with teachers in India. We’ve tested this with them. The teachers are very excited and now Sikshana wants to scale it to other schools.
HUIZINGA: Right … well, my first question is, where were you when I was teaching, Kalika?
BALI: There was no generative AI!
HUIZINGA: No. In fact, we just discovered the fax machine when I started teaching. Oh, that dates me! You know, back to what you said about teachers being instrumental in the lives of their students. You know, we can remember our favorite teachers, our best teachers. We don’t remember a machine.
BALI: No.
HUIZINGA: And what you’ve done with this is to embody the absolute sort of pinnacle of what AI can do, which is to be the collaborator, the assistant, the augmenter, and the helper so that the teacher can do that inspirational, connective-tissue job with the students without having to, like, sacrifice the rest of their life making lesson plans and grading papers. Oh, my gosh. OK. On the positive side, we’ve just talked about what this work proposes and how it’s good, but I always like to dig a little bit into the potential unintended consequences and what could possibly go wrong if, in fact, you got everything right. So I’ll anchor this in another example. When GPT models first came out, the first reaction came from educators. It feels like we’re in a bit of a paradigm shift like we were when the calculator and the internet even came out. [It’s] like, how do we process this? So I want to go philosophically here and talk about how you foresee us adopting and moving forward with generative AI in education, writ large.
BALI: Yeah, I think this is a question that troubles a lot of us and not just in education, but in all spheres that generative AI is …
HUIZINGA: Art …
BALI: … art …
HUIZINGA: … writing …
BALI: … writing …
HUIZINGA: … journalism …
BALI: Absolutely. And I think the way I, kind of, think about it in my head is it’s a tool. At the end of it, it is a tool. It’s a very powerful tool, but it is a tool, and humans must always have the agency over it. And we need to come up, as a society, you know, we need to come up with the norms of using the tool. And if you think about it, you know, internet, taking internet as an example, there is a lot of harm that internet has propagated, right. The darknet and all the other stuff that happens, right. But on the whole, there are regulations, but there are also an actual consensus around what constitutes the positive use of internet, right.
HUIZINGA: Sure, yeah.
BALI: Nobody says that, for example, deepfakes are …
HUIZINGA: Mm-hmm. Good …
BALI: … good, right. So we have to come from there and think about what kind of regulations we need to have in place, what kind of consensus we need to have in place, what’s missing.
HUIZINGA: Right. Another project that has been around, and it isn’t necessarily on top of VeLLM, but it’s called Karya, and you call it a social impact organization that serves not just one purpose, but three. Talk about that.
BALI: Oh, Karya is my favorite! [LAUGHS] So Karya started as a research project within Microsoft Research India, and this was the brainchild again of my colleague—I have like some of the most amazing colleagues, too, that I work with!—called Vivek Seshadri. And Vivek wanted to create, you know, digital work for people who do not have access to such work. So he wanted to go to the rural communities, to people who belong to slightly lower socioeconomic demographies, and provide work, like microtasks kind of work, gig work, to them. And he was doing this, and then we started talking, and I said, you know, we need so much data for all these languages and all these different tasks, and that could be, like, a really cool thing to try on Karya, and that’s where it all started, my involvement with Karya, which is still pretty strong. And Karya then became such a stable project that Microsoft Research India spun it out. So it’s now its own standalone startup right now like a social enterprise, and they work on providing digital work. They work on providing skills, like upskilling. They work on awareness, like, you know, making people aware of certain social, financial, other such trainings. So what’s been most amazing is that Karya has been able to essentially collect data for AI in the most ethical way possible. They pay their workers a little over the minimal wage. They also have something called data ownership practice, where the data that is created by, say, me, I have some sort of ownership on it. So what that means is that every time Karya sells a dataset, a royalty comes back …
HUIZINGA: No … !
BALI: Yeah! To the workers.
HUIZINGA: OK, we need to scale this out! [LAUGHS] OK. So to give a concrete example, the three purposes would be educational, financial—on their end—and data collection, which would ultimately support a low-resource language by having digital assets.
BALI: Absolutely!
HUIZINGA: So you could give somebody something to read in their language …
BALI: Yeah.
HUIZINGA: … that would educate them in the process. They would get paid to do it, and then you would have this data.
BALI: Yes!
HUIZINGA: OK. So cool. So simple.
BALI: Like I said, it’s my favorite project.
HUIZINGA: I get that. I totally get that.
BALI: And they … they’ve been, you know, they have been winning awards and things all over for the work that they’re doing right now. And I am very involved in one project with them, which is to do with gender-intentional AI, or gender-intentional datasets for AI, for Indian languages. And that’s really crucial because, you know, we talk about gender bias in datasets, etc., but all that understanding comes from a very Western perspective and for languages like English, etc. They do not translate very well to Indian languages.
HUIZINGA: Right.
BALI: And in this particular project, we’re looking at first, how to define gender bias. How do we even get data around gender bias? What does it even mean to say that technology is gender intentional?
HUIZINGA: Right. All right, well, let’s talk a little bit about what I like to call outrageous ideas. And these are the ones that, you know, on the research spectrum from sort of really practical applied research to blue sky get dismissed or viewed as unrealistic or unattainable. So years ago—here’s a little story about you—when you told your tech colleagues that you wanted to work with the world’s most marginalized languages, they told you you’d only marginalize yourself.
BALI: Yes!
HUIZINGA: But you didn’t say no. You didn’t say no. Um, two questions. Did you feel like your own idea was outrageous back then? And do you still have anything outrageous yet to accomplish in this plan?
BALI: Oh, yeah! I hope so! Yeah. No, I do think, in some sense, the pushback that I got for my idea makes me think it was outrageous. I didn’t think it was outrageous at all at that time! [LAUGHS] I thought it was a very reasonable idea! But there was a very solid pushback and not just from your colleagues. You know, for researchers, publishing papers is important! No one would publish a paper which focused only on, say, Indian languages or low-resource languages. We’ve come a very long way even in the research community on that, right. We kept pushing, pushing, pushing! And now, there are tracks, there are workshops, there are conferences which are devoted to multilingual and low-resource languages. When I said I wanted to work on Hindi, and Hindi is the biggest language in India, right. And even for that, I was told, why don’t you work on German instead? And I’m like, there are lots of people working on German who will solve the problems with German! Nobody is looking at Hindi! I mean, people should work on all the languages. People should work on German, but I don’t want to work on German! So there was a lot of pushback back then, and I see a little bit of that with the very low-resource languages even now. And I think some people think it’s a “feel-good” thing, whereas I think it’s not. I think it’s a very economically viable, necessary thing to build technology for these communities, for these languages. No one thought Hindi was economically viable 15 years ago, for whatever reason …
HUIZINGA: That … that floors me …
BALI: Yeah, but, you know, we’re not talking about tens of thousands of people in some of these languages; we’re talking about millions.
HUIZINGA: Yeah.
BALI: I still think that is a job that I need to continue, you know, pushing back on.
HUIZINGA: Do you think that any of that sort of outrageous reaction was due to the fact that the technology wasn’t as advanced as it is now and that it might have changed in terms of what we can do?
BALI: There was definitely the aspect of technology there that it was just quite difficult and very, very resource-intensive to build it for languages which did not have resources. You know, there was a time when we were talking about how to go about doing this, and because people in various big tech companies, people did not really remember a time when, for English, they had to start data collection from scratch because everyone who was working on, say, English at that time was building on what people had done years and years ago. So they could not even conceptualize that you had to start from scratch for anything, right. But now with the technology as well, I’m quite optimistic and trying to think of how cool it would be to do, you know, smaller data collections and fine-tuned models specifically and things like that, so I think that the technology is definitely one big thing, but economics is a big factor, too.
HUIZINGA: Mmm-hmm. Well, I’m glad that you said it isn’t just the feel good, but it actually would make economic sense because that’s some of the driver behind what technologies get “greenlit,” as it were. Is there anything outrageous now that you could think of that, even to you, sounds like, oh, we could never do that …
BALI: Well … I didn’t think HAL was outrageous, so I’m not … [LAUGHS]
HUIZINGA: Back to HAL 9000! [LAUGHS]
BALI: Yeah, so I don’t think of things as outrageous or not. I just think of things as things that need to get done, if that makes any sense?
HUIZINGA: Totally. Maybe it’s, how do we override “Open the pod bay door, HAL”—“No, I’m sorry, Dave. I can’t do that”? [LAUGHS]
BALI: Yes. [LAUGHS] Yeah…
HUIZINGA: Well, as we close—and I’m sad to close because you are so much fun—I want to do a little vision casting, but in reverse. So let’s fast-forward 20 years and look back. How have the big ideas behind your life’s work impacted the world, and how are people better off or different now because of you and the teams that you’ve worked with?
BALI: So the way I see it is that people across the board, irrespective of the language they speak, the communities they belong to, the demographies they represent, can use technology to make their lives, their work, better. I know it sounds like really a very big and almost too good to be true, but that’s what I’m aiming for.
HUIZINGA: Well, Kalika Bali, I’m so grateful I got to talk to you in person. And thanks for taking time out from your busy trip from India to sit down with me and our audience and share your amazing ideas.
[MUSIC PLAYS]
BALI: Thank you so much, Gretchen.
[MUSIC FADES]